THE CONTINUED IMPORTANCE OF MICROBICIDES IN INDUSTRIAL APPLICATIONS
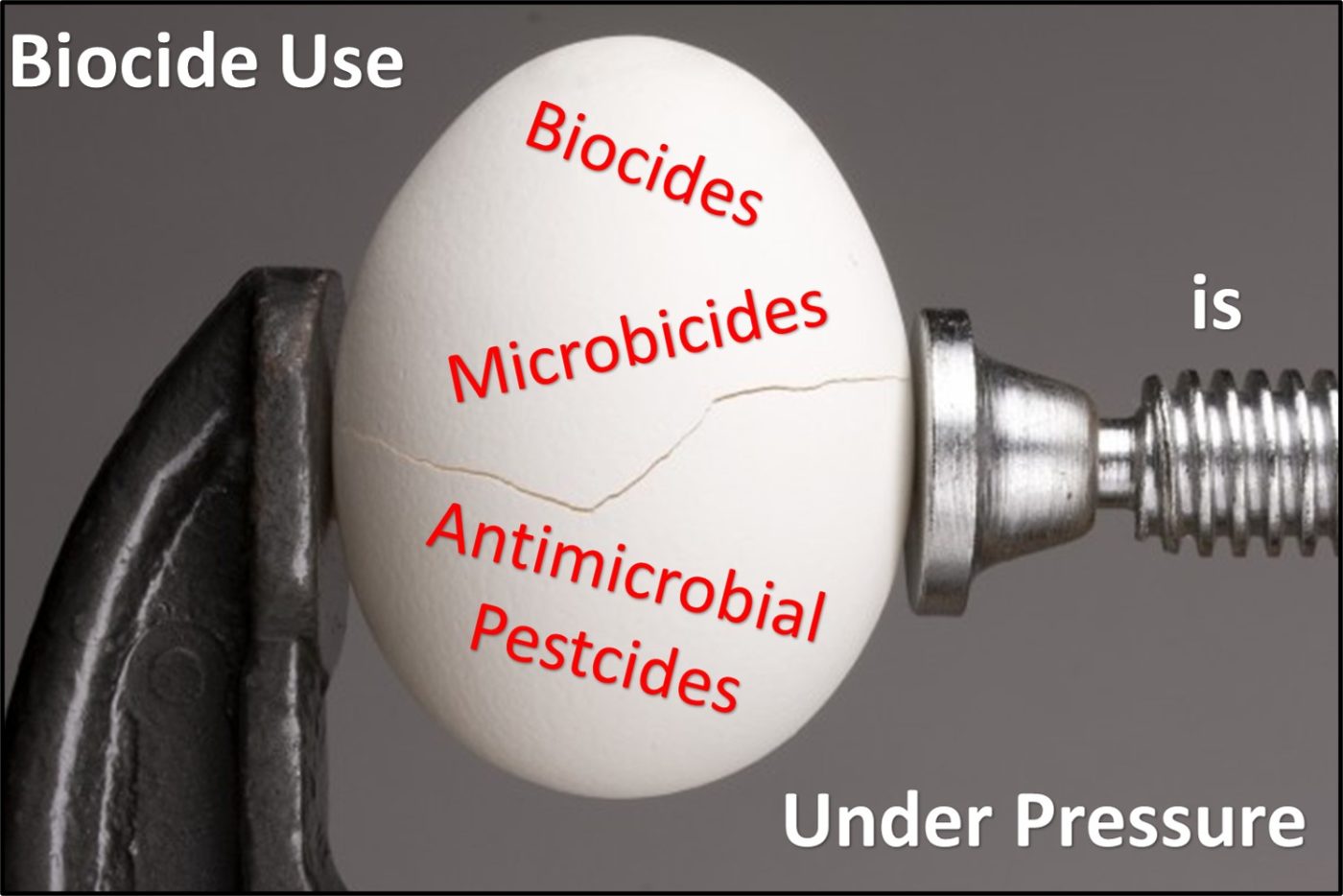
The Challenge
At this year’s STLE Annual Meeting I presented a paper titled: “The continued importance of antimicrobial pesticides for controlling microbial contamination in industrial process fluids and systems.” Subsequently, the editors of Lube Magazine (https://www.lube-media.com/) invited me to write an article on the topic. That article – available from the Lube Media website only to Lube Magazine subscribers – was published in Lube Magazine, Issue 181, June 2024. I’ve also archived the article here on BCA’s website.
The crux of the issue is that for the past several decades, regulatory agencies have been increasingly myopic regarding the assessment of risks associated of biocidal products. The net effect has been a shrinking number of microbicidal active substances approved for use in Canada, Europe, the U.S., and other countries where microbicide use is regulated. The number of toxicological and environmental tests required to support new-product and registration renewal applications continues to grow as do end-use restrictions. For example, in 2000, the average cost for toxicological testing in support of industrial microbicide applications was less than $200,000 U.S. As of 2022, that average cost was between $1 million and $2 million U.S. The total global market for microbicides used in metalworking fluids (MWF) is estimated at $300 million U.S. Consequently, the potential return on investment no longer justifies the development of new active substances for controlling microbial contamination in MWF. Even the initial investment in toxicity testing to support product registrations is insufficient. All registered biocidal products are reviewed periodically for reregistration eligibility. In their 27 June 2008 Reregistration Eligibility Document (RED) for Hexahydro-1,3,5tris(2hydroxyethyl)-s-triazine (triazine), the U.S. EPA limited the maximum end-use dosage to 500 ppm – a dosage that’s less than the minimum concentration needed for triazine to work effectively. U.S. EPA’s OPP cited their concerns over formaldehyde exposure risk and their (incorrect) understanding of a 2023 paper.
Regulatory Agencies and Regulations
Table 1 lists selected regulatory agencies responsible for pesticide use oversight in the EU and specific countries, and the regulations under which the different agencies function. Within the EU, biocidal products approved by ECHAs Biocidal Products Committee (BPC) must also be approved the cognizant agencies of each country in which the products are sold. As for all products regulated under REACH, biocidal products are assessed based on their hazard (toxicological properties) rather than the risks associated with their use. Putatively, in the U.S., the U.S. EPA’s Office of Pesticide Programs (OPP) focuses on risk (the likelihood that users will suffer injuries). However, recent RED’s illustrate how OPP administrators tend to allow their understanding of hazard to trump the risk assessment (more on this below).
Table 1. National and Regional Agencies and Regulations Administering Microbicide Use.
Canada | Pest Control Products Act (PCPA) | Pest Management Regulatory Agency (PMRA) |
E.U. | Biocidal Products Regulation (BPR) | Biocidal Products Committee (BPC) |
India | Guidelines for the Registration of Biocide and Biocide Products | Central Insecticide Board and Registration Committee (CIB&RC) |
Japan | Chemical Substances Control Law (CSCL) | Ministry of Economy, Trade, and Industry (METI) |
People’s Republic of China | New Pesticide Management Regulation | Institute for the Control of Agrochemicals, Ministry of Agriculture (ICAMA) |
U.S. | Federal Insecticide, Rodenticide, and Fungicide Act | U.S. Environmental Protection Agency, Office of Pesticides Programs (U.S. EPA, OPP) |
Note: a) This list illustrates the fact that globally there are numerous agencies responsible for regulating biocide use. It is not meant to be exhaustive.
The list of animal and ecotoxicological tests required varies by agency. In the U.S. an initial, relatively short list of toxicological tests are required in support of a pesticide application. The OPP representative reviewing the application can request additional tests. It is common for applicants to spend years incrementally supplying OPP with additional test results. Rather than either rejecting an application or providing the applicant with a compete list of additional tests that must be performed, OPP application reviewers tend to request additional tests, one at a time. For one product of which I am aware, the application for OPP approval for a product to be used in MWF has been going on for more than 15 years. In other instances, microbicide manufacturers had opted to withdraw heir products from the market rather than spend $ millions on additional toxicity, environmental fate and persistence, or both types of tests required in support of REDs. As a result, the number of microbicides available for use in MWF in the U.S. is <30 % of the number that were available in 1995. Similarly, in the EU, only 27 active substances are currently approved for use in MWF. That list was >200 in 1995.
Factors Driving Increased Regulatory Pressure
Hazard and risk conceptual conflation
You have no doubt seen variations of the meme in which a character is so focused on a relatively minor hazard that they are oblivious to a major one (Figure 1). A person backs away from a mouse and over a cliff, person is so focused on a bear cub that they remain unaware of the mama bear that’s about to attack – the examples go on. The issue is the same for the human tendency to conflate hazards with risks.
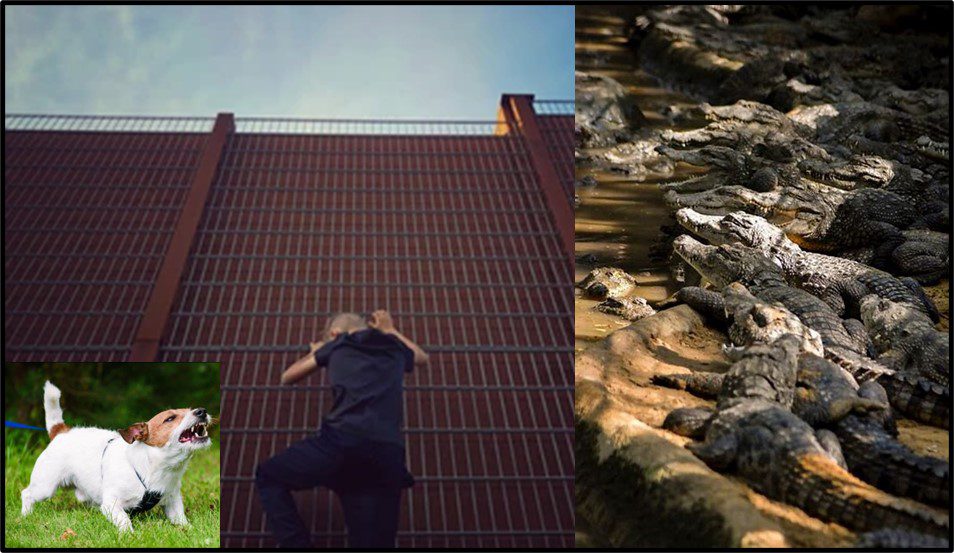
Fig 1. From the frying pan into the fire – person climbing the fence to escape the growling (petite) dog will undoubtedly not like the crocodiles waiting for him once he climbs over.
As I noted above, risk (R) is the likelihood (probability) that harm will occur. It is a function of hazard (H) and exposure (E), where hazard is the severity of harm likely to occur when the agent is contact, and exposure the degree of contact:
R = H x E (1)
For chemical substances, hazard is assessed based on the doses at which different types of toxicological damage occur. Dosage for direct contact or ingestion is reported in mg of test substance per kg of test organism body weight (mg kg-1). For inhalation studies, it is reported as mg L-1 in the air the test organisms breath. Most commonly, the hazard measured is death, and the end point is the test substance concentration that kills half of the exposed population (lethal dose 50 – LD50 or lethal concentration – LC50). The acute tests that are required universally include:
- Oral toxicity
- Eye irritation
- Dermal toxicity
- Skin irritation
- Inhalation toxicity
- Skin sensitization
Other endpoints include tissue damage, impact on the test organisms’ metabolism, and other pathological symptoms. Acute toxicity tests measure the effect of a single exposure. Sub-chronic toxicity tests measure the effect of repeated exposures during a one-month test period. Chronic toxicity tests evaluate the test substance’s impact during the life of the test organism.
A chemical substance’s toxicity depends on its route of exposure. Figure 2a compares the acute oral toxicities of two chemicals I’ve designated as A and B. Figure 2b compares the acute dermal toxicities for the same two chemicals. Note that for oral toxicity, chemical A is more toxic than chemical B, but that for inhalation toxicity, B is more toxic than A. For chemical A, the oral LD50 = 200 mg kg-1 and the dermal LD50 is 1,000 mg L-1. Conversely, chemical B’s is more toxic through dermal contact than it is via the oral route.
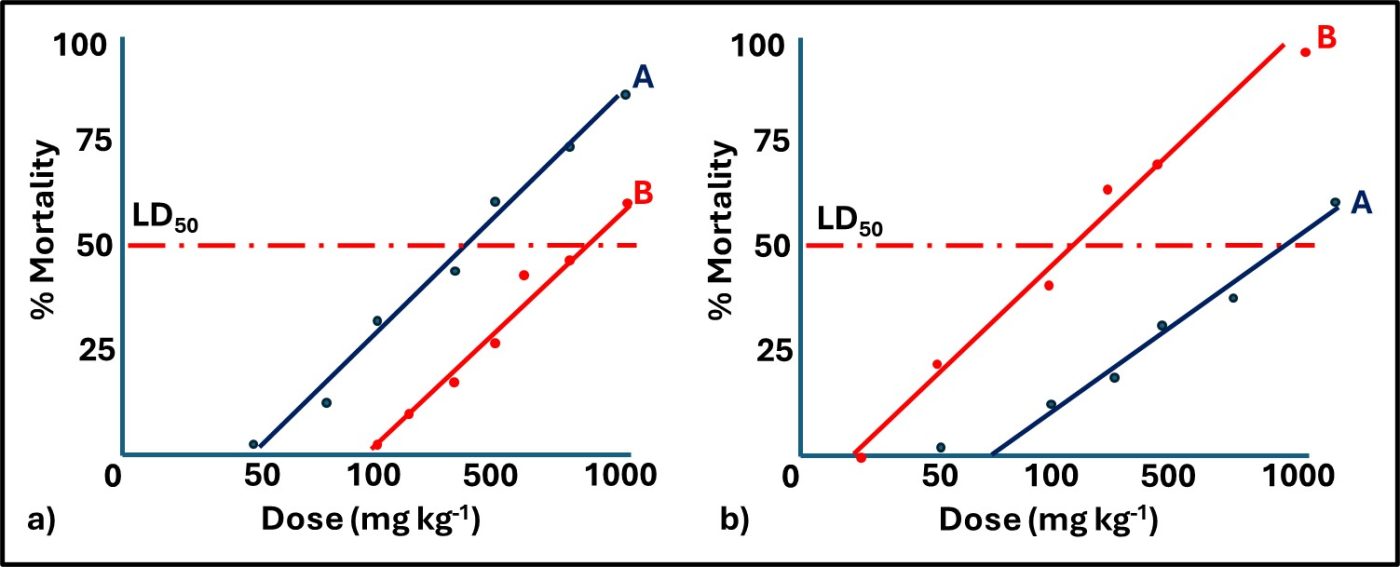
Fig 2. Acute toxicity dose response curves – a) oral toxicity: substance A’s and B’s LD50 = 200 mg kg-1 and 750 mg kg-1, respectively; b) dermal toxicity: substance A’s and B’s LC50 = 1000 mg kg-1 and 100 mg kg-1, respectively.
In Figures 1a and 1b, none of the trend lines intersect with the Dose axis at 0 mg kg-1 or 0 mg L-1. The doses at which the trend lines intersect the Dose axis is called the no observable effect level (NOEL). Biological modeling plots toxicity test results to show the NOEL. Linear modelling forces the trend line through the origin (i.e., 0 % mortality at 0 mg kg-1 test substance concentration). If the four trendlines in Figure 1 were adjusted to go through the origin both test substances would appear to be more toxic than they actually are. The U.S. EPA uses the linear model to assess test substance toxicity. In other words, they tend to overestimate the hazard.
The second factor affecting risk is exposure. Exposure is a function of a substance’s concentration and the period of exposure. Being struck by a moving car is a hazard. The risk of being struck depends on the number of cars passing the exposed individual per unit of time (e.g., minute). In terms of cars passing per minute, in Figure 3 the couple walking on a country lane are exposed to a fraction of the cars to which the crowd walking on the freeway are. In industrial settings, without appropriate industrial hygiene controls in place (personal protective equipment, ventilation, etc.) workers handling concentrated chemicals potentially experience greater exposure than do workers exposed to diluted chemicals. However, when the workplace complies with hazardous material handling regulations, worker exposure is minimized.
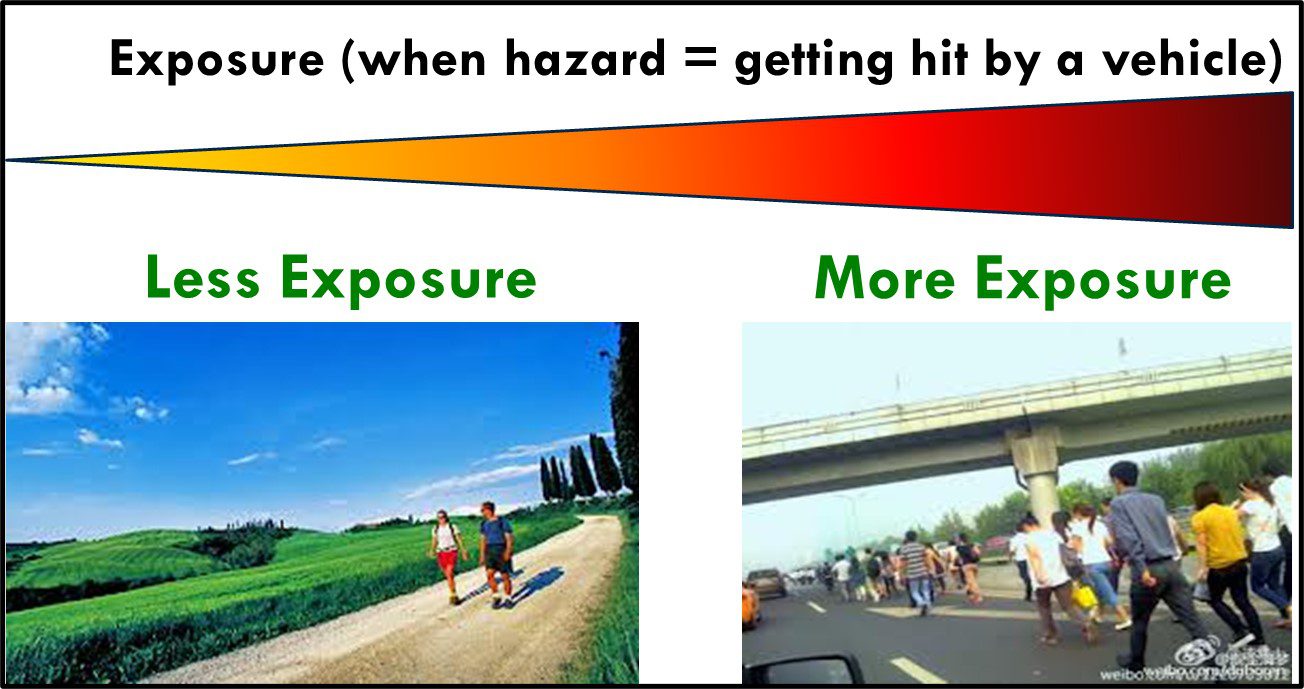
Fig 3. Exposure – the couple on the left, walking on a country lane, are exposed to far fewer cars than are the people walking into a traffic-dense highway.
According to the Governors Highway Safety Association (GHSA), there were 7,485 pedestrians killed by moving vehicles in 2021. I’ll leave it for my readers to speculate how that statistic compares with the total number of industrial workers who have been killed by microbicide exposure in the past 100 years. When injuries have occurred, the cause has invariably failure to follow manufacturer’s handling instructions. The number of injuries associated with accidental exposure to non-biocidal chemicals is much greater than the number related to biocide exposure.
Narrow risk focus
Microbicides serve an important function. They are designed to control microbial contamination – they kill microorganisms. Globally, damage caused by microorganisms is estimated to cost hundreds of $ billions annually. These costs include spoilage (food, process fluids, specialty chemicals, paints and coatings, personal care products and countless other biodegradable materials), structural damage (primarily microbiologically influenced corrosion and biofouling), and health effects (allergies, toxemias, and infectious diseases). Microbicides play an essential role in reducing the health risks and other costs associated with microorganisms. However, regulatory agencies rarely consider the risks associated with not controlling microbiological contamination.
The formaldehyde issue
Currently, the U.S. EPA has targeted all formaldehyde-condensate microbicides for elimination. Their logic is:
Argument 1: Formaldehyde is a carcinogen.
Argument 2: Formaldehyde-condensate microbicides are manufactured by reacting formaldehyde with one or more other chemicals.
Argument 3: The mode of action of most formaldehyde-condensate microbicides is to release formaldehyde so that it can react with microorganisms’ structural and enzyme proteins.
Argument 4: If formaldehyde is released when formaldehyde-condensate microbicides kill microorganisms, it must also be released when those microbicides are diluted into treated fluids (e.g., metalworking fluids, paints, personal care products, etc.).
Therefore, people using formaldehyde-condensate microbicides are exposed to formaldehyde.
Unfortunately, this logic is flawed. The facts do not support argument 4. Modern analytical chemistry testing on fluids treated with formaldehyde-condensate microbicides have demonstrated that if any free-formaldehyde is present, it is at concentrations below detection levels (i.e., <1 μg kg-1 – ppb). Moreover, studies to test for formaldehyde in the air above formaldehyde-condensate microbicide treated and untreated metalworking fluids have determined that statistically there is no difference.
There’s a second flaw in the logic. The EPA recognizes that living beings produce formaldehyde. Except for the studies showing that formaldehyde concentrations in the air above untreated metalworking fluids were in the same range as above treated fluids, no research has reported the impact of uncontrolled microbial contamination on formaldehyde. Studies have also demonstrated that only formaldehyde-condensate microbicides denature endotoxins produced by Gram-negative bacteria that commonly grow in metalworking fluids. These last two considerations suggest that if formaldehyde-condensate microbicides can no longer be used in metalworking fluids, worker health risks due to endotoxin and other biological components (bioaerosols) would be greater than it is at present. It would be like rolling the microbial contamination control clock back to the 1960s when operators never had microbiological problems. Back then they only had odor problems.
In 1996, Lubrication Engineering published my paper: “Formaldehyde Risk in Perspective: A Toxicological Comparison of Twelve Biocides.” In that paper, I compared toxicity date for formaldehyde and a dozen MWF microbicides. The list included formaldehyde-condensates and nominally formaldehyde-free microbicides. In most test categories, the microbicidal products were less toxic than formaldehyde. None were either mutagenic or carcinogenic. Toxicity was not related to the presence of formaldehyde as a reactive intermediate.
The U.S. EPA has not been deterred by these facts. It is likely that formaldehyde-condensates will no longer be approved for use in MWF or other industrial applications.
Hysteria versus science
Equation 1 illustrated the relationship between hazard, risk, and exposure. It does not include the term that often overwhelms scientific assessment. That term is outrage. Outrage is the reaction individuals have to what they perceive to be unacceptable risk. This acceptability assessment is purely subjective. Risks that some people find totally acceptable, others find intolerable. Intolerance leads to outrage. Despite the well documented risks linked to smoking, the population of smokers consider the risk to be acceptable. Some non-smokers respond to secondary smoke exposure with outrage. Similarly, despite the nuclear power industry’s remarkable safety record, opponents of nuclear energy commonly react to proposed nuclear power generation facility construction proposals with outrage. Invariably, hard data are discarded when outrage appears. For biocides, it is the word itself rather than biocidal product toxicity that triggers outrage.
The words “biocide” or “microbicide” stimulate fear and anxiety even when the product’s toxicological data are more benign than those of other industrial chemicals. For example, in recent years a growing number of MWF compounders have been promoting biocide-free products. Some have been replacing traditional microbicides with an amine – dicyclohexylamine (DCHA). To date, the only published DCHA performance data demonstrate that it prevents uncontrolled microbial contamination from developing in MWF. Nothing has been reported about DCHA’s performance as a corrosion inhibitor, pH buffer, or other non-biocidal, functional additive. Figure 4 compares the acute oral, dermal, and inhalation toxicities of DCHA and hexahydro-1,3,5-tris(2-hydroxyethyl)-s-triazine (triazine – HHT). DCHA’s acute oral toxicity is 7x greater than HHT’s and its dermal toxicity is >10x that of HHT. Although HHT has a record of >70 years of safe, effective use, it is being replaced by a more toxic product that does not have a pesticide registration.
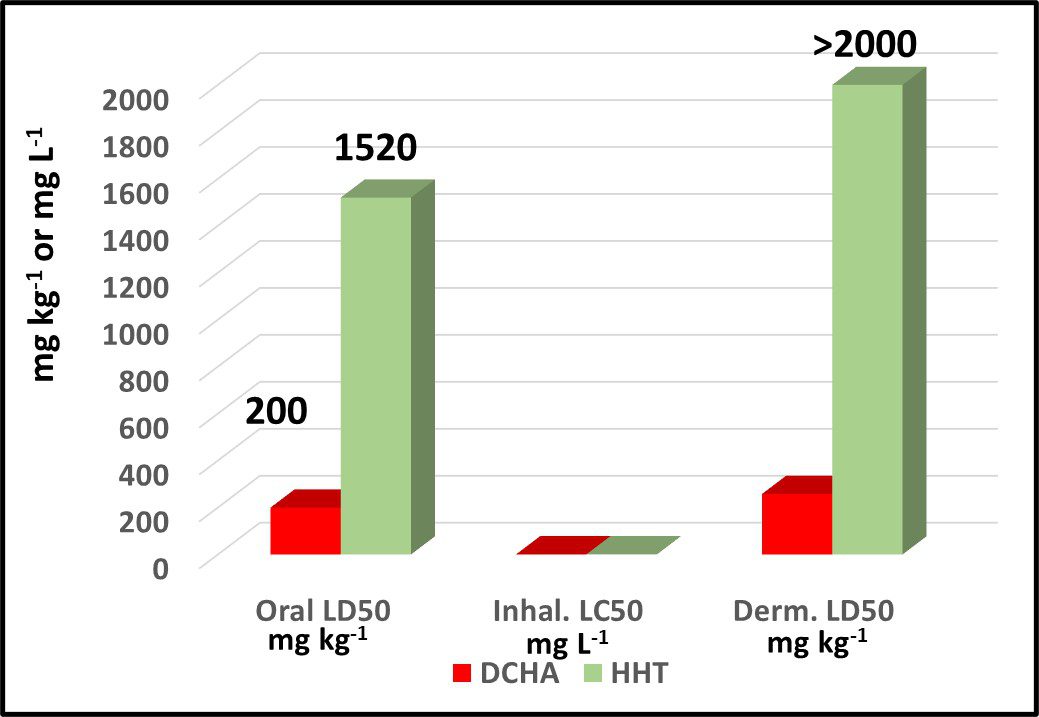
Fig 4. Toxicity comparison – dicyclohexylamine (DCHA) and hexahydro-1,3,5-tris(2-hydroxyethyl)-s-triazine (HHT).
A Rational Path Ahead
I have a simple proposition. Level the playing field. I suggest that governmental agencies responsible for industrial chemicals revise their regulations so that all industrial chemicals have the same data requirements for approval. Countless industrial chemicals are more toxic than those registered as biocidal products. Currently, the toxicological and environmental data available for many non-biocidal products are substantially more limited than data for biocidal products. Similarly, for a given chemical that has technical and biocidal uses, the amount of information required on a biocidal product’s label is substantially greater than on a technical product’s label (Figure 5).
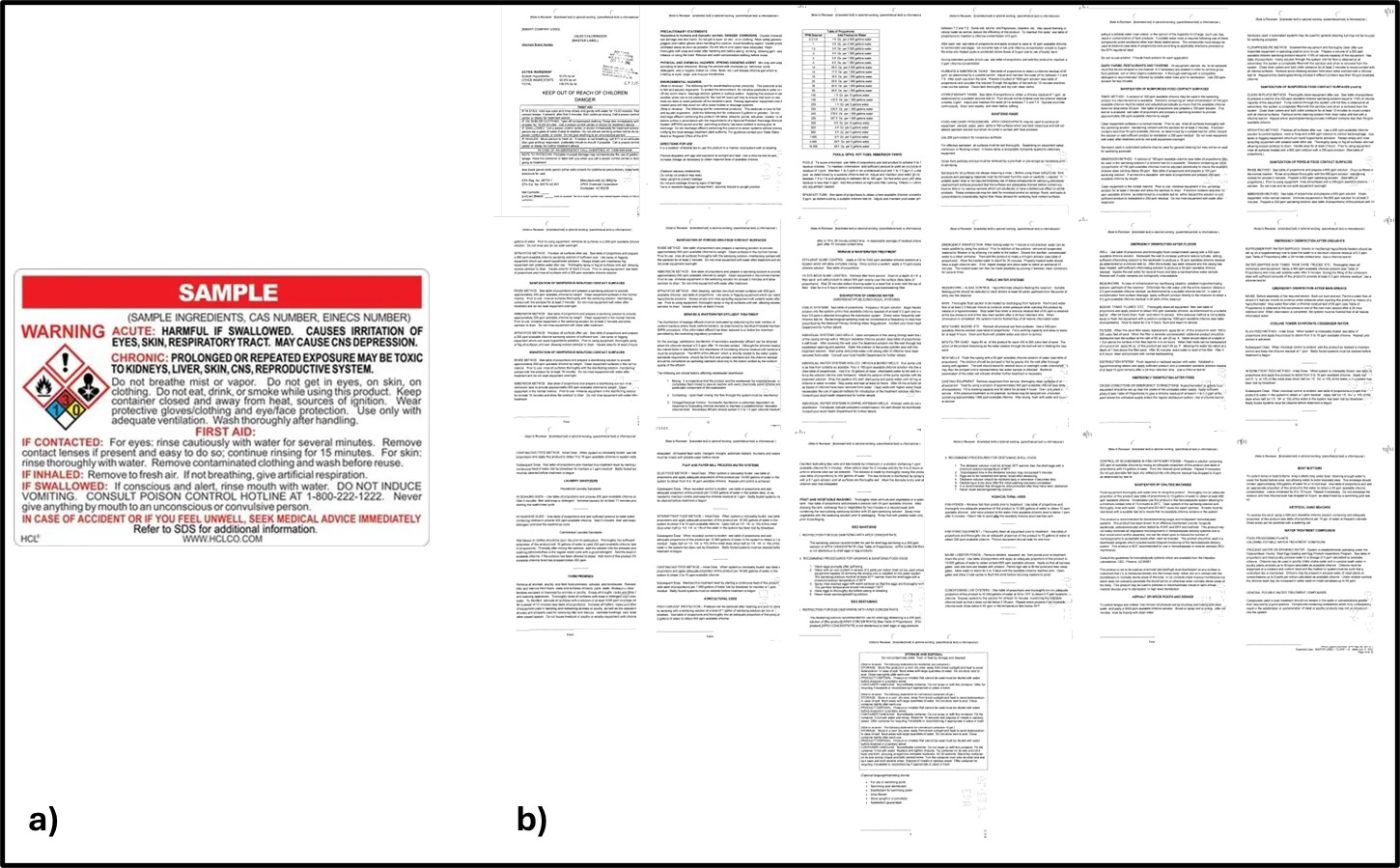
Fig 5. Product labels for 10 % Sodium Hypochlorite (NaOCl – bleach) – a) label on household bleach bottle; b) master label for 10 % NaOCl as an algicide (22 pages).
Chemical substances’ adverse health effects are typically discovered only years after the general population has been exposed. If all products were required to be supported by the same toxicity and environmental data, manufacturers would lose their incentive to replace approved biocidal products with potentially more toxic substitutes.
Summary
Microbicides are under increasing regulatory pressure. This pressure translates into an increased number of tests requires in support of registration and reregistration applications. The additional tests are substantially more expensive than those run for technical grade chemicals and typically add up to more than $2 million U.S. These costs have a chilling impact against new microbicide development. The greater toxicity testing burden for biocidal products does not reflect either increased exposure risk or chemical hazard. It is based on hysterical reactions to the word biocide. The unintended consequences of this trend will be increased health risks and biodeterioration costs.
I look forward to your thoughts and questions about how industry stakeholders can reverse this shrinking availability of biocidal products. Contact me at fredp@biodeterioration-control.com.